Trustworthy Interaction Between Automated Vehicles and Pedestrians
Understanding and improving pedestrian trust in automated vehicles through behavioral studies and predictive models for safe, interpretable AV-pedestrian interactions.
This project area focuses on building trustworthy interactions between automated vehicles (AVs) and pedestrians, focusing on pedestrian perception of trust in automated vehicles. A major challenge is handling ambiguous right-of-way situations, such as unsignalized crosswalks.
A human subjects study using an Immersive Virtual Environment (IVE) and a pedestrian walking simulator revealed that aggressive AV driving behavior significantly reduced pedestrian trust, while signalized crosswalks mitigated this effect. Notably, higher trust correlated with riskier pedestrian behavior, such as jaywalking and reduced gaze toward AVs, raising concerns about overtrust.
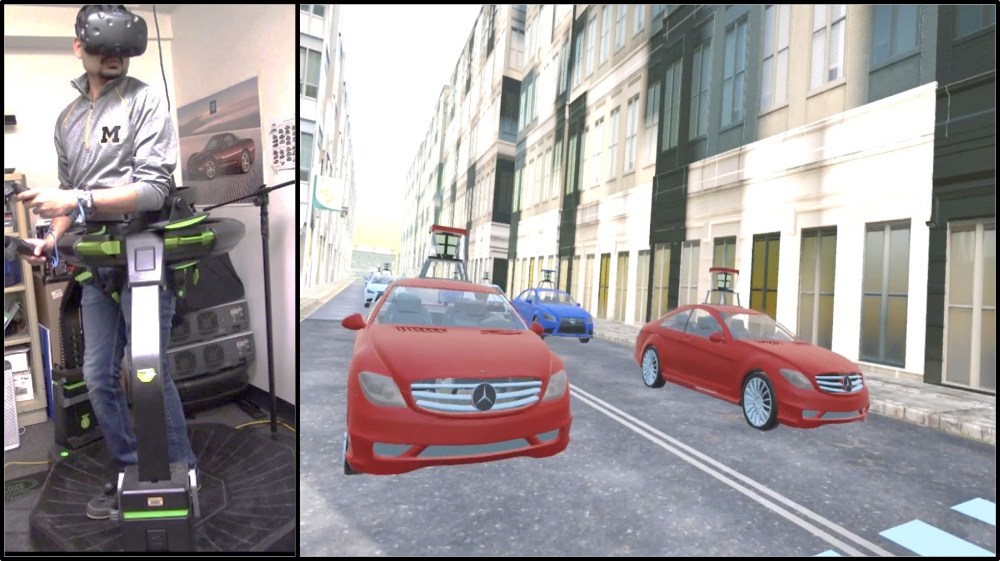
To validate findings in a real-world setting, a follow-up study was conducted at the Mcity test track using a Wizard-of-Oz AV and a human-driven vehicle (HDV). Surveys confirmed that pedestrians initially trusted HDVs more than AVs, but trust in AVs increased more with repeated interactions. This suggests that familiarity can help reduce skepticism and improve AV-pedestrian relations over time. A key insight from the study was that pedestrians considered HDVs to be more aggressive than AVs, yet they still trusted HDVs more. This suggests that pedestrians may be more forgiving of aggressive behavior from human drivers compared to AVs. It also indicates that pedestrians expect AVs to be less aggressive and more conservative than HDVs
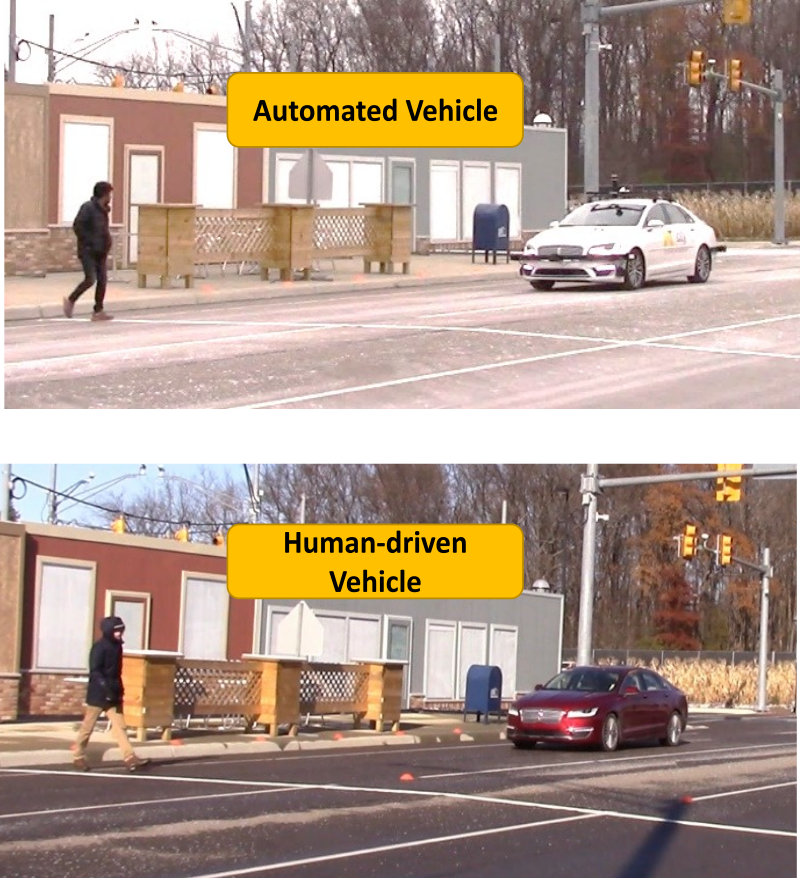
To support safer interactions, we developed pedestrian behavior prediction models like the Multimodal Hybrid Pedestrian (MHP) model. These models feed into behavior-aware control strategies (e.g., B-MPC), enabling AVs to anticipate pedestrian intent and plan less conservative, yet safer, paths. Such predictive planning allows AVs to act in ways that pedestrians can interpret and trust, improving communication and cooperation in shared urban spaces.